Due to the decrease in commodity prices in a constantly dynamic environment, there has been a constant urge to maximize benefits and attain value from limited resources. Traditional empirical and numerical simulation techniques have failed to provide comprehensive optimized solutions in a relatively short time. Coupled with the immense volumes of data generated daily, a solution to tackle industry challenges became imminent. Various expert opinion fraught with bias has posed extra challenges to obtain timely cost-effective solutions. Data Analytics has provided substantial contributions in several sectors. However, its full potential value has not been captured in the Oil and Gas industry yet.
Artificial Intelligence (AI) is the fascinating field of combining human intelligence and computing power in generating smart or intelligent solutions to complex problems. AI based solutions are capable of not only simulating human brain functionality but are also adept at using the intelligent algorithms to understand the problem and provide solutions. Ever since the early 1980s, AI has been integrated in various business applications. Literature survey shows that AI has been spreading in all industrial applications and providing fast, reliable and easy to use solutions to complex problems in this time of Fourth Industrial Revolution (4IR). Interestingly, the areas of research in AI has been growing many folds, with techniques penetrating new avenues and industrial applications.
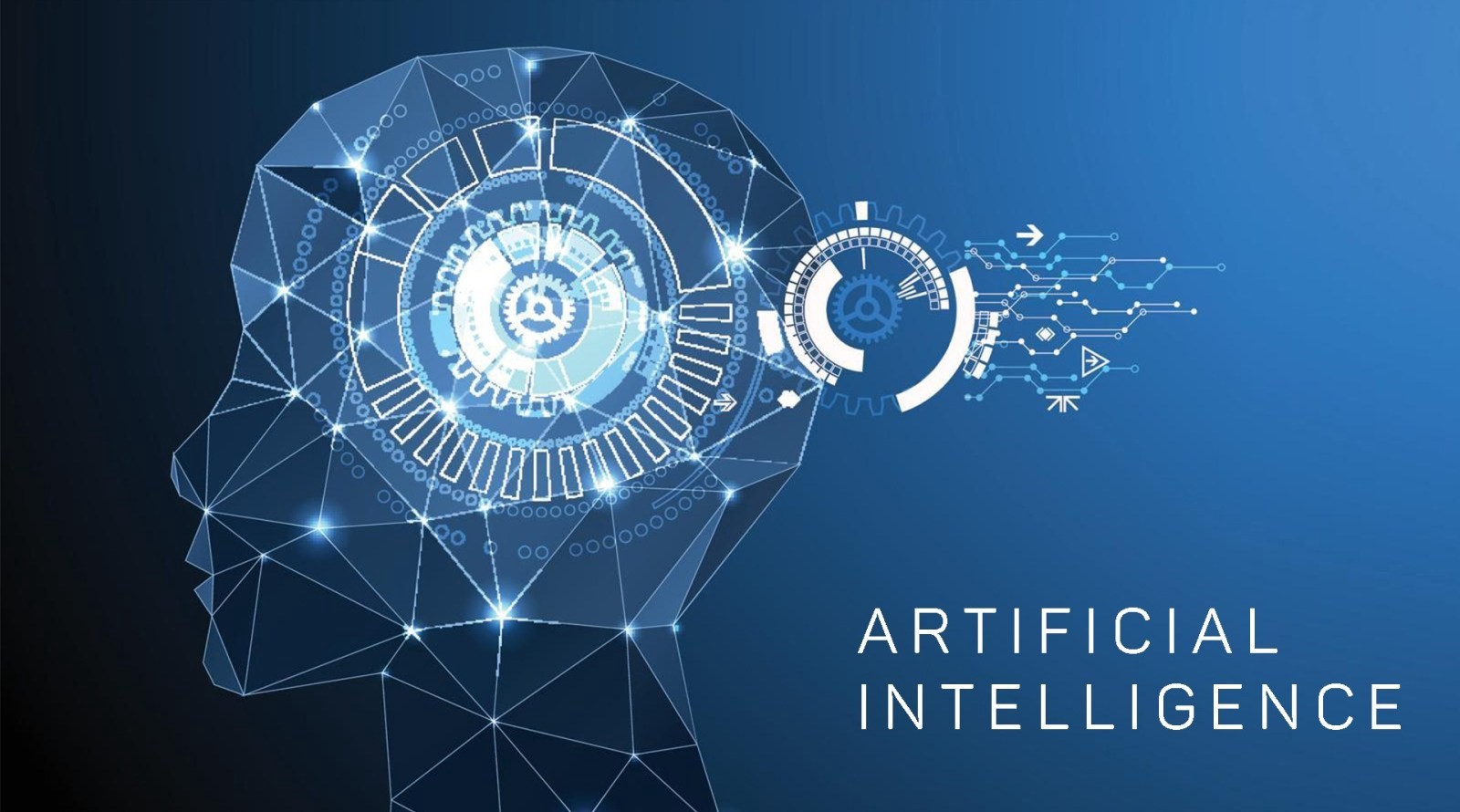
Application of AI at CPG has been very active in the recent past. A number of problems have been addressed in the area of petroleum engineering applications. These include porosity-permeability predictions, hydraulic flow unit identification, PVT parameters estimation, geomechanics parameters estimation, drilling parameters estimation, geophysical well logs estimation, hydraulic fracturing performance evaluation, field surveillance, production management, well test parameters estimation, asphaltene prediction, water saturation prediction, and a number of other areas.
A team led by Dr. Abdulazeez Abdulraheem, his colleagues and students executed a research project at CPG titled as “Prediction of PVT properties”. Characterization of reservoir fluids plays a very crucial role in developing strategy on how to produce and operate a reservoir. Pressure-Volume-Temperature (PVT) Properties are very important input for geophysics and petroleum engineers, namely for the utilization in material balance calculations, well log analysis, inflow performance calculations, determining oil reserve estimations and the amount of oil that can be recovered, the flow rate of oil or gas and the simulations on reservoir outputs.
To predict PVT properties, several AI tools were used. These include Support Vector Regression (SVR), Extreme learning machines (ELM), Radial Basis Function NN (RBF), Multi level Non-Linear Regression (MLNLR), Forward Functional Network (FN), Fuzzy Logic (FL), and Artificial Neural Network (ANN).
Based on the composition of different oil types, successful predictions were made for Flash Test, Compressibility, Differential Test, Viscosity Test, and Separator Test using the AI tools.
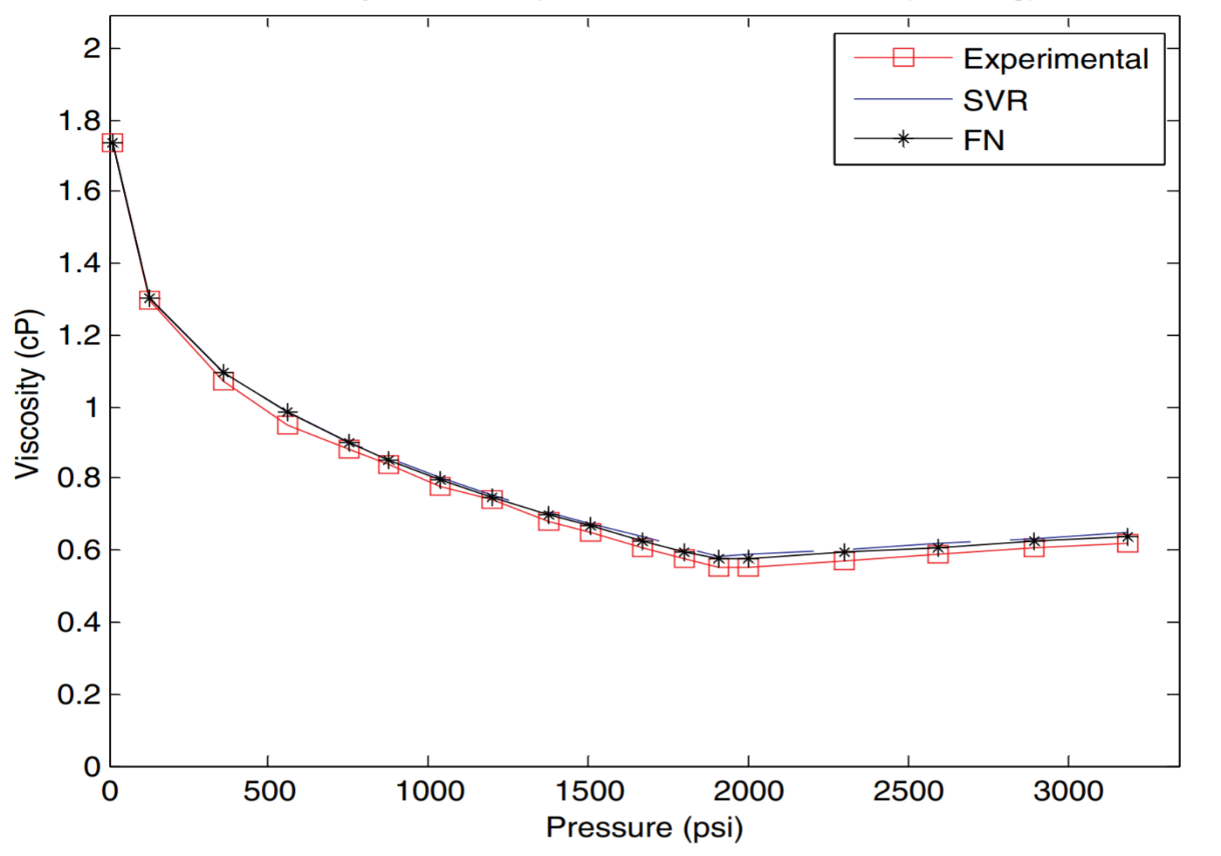
Figure: Viscosity test (Prediction vs. experimental data for viscosity vs. pressure plot for a typical sample using SVR and FN)
The team at CPG includes Dr. Abdulazeez Abdulraheem, Dr. Saleh Elkatatny, Dr. Mohammed Mahmoud, Mr. Zeeshan Tariq, and Mr. AbdulMalek Saif Ahmad.
References:
- Khoukhi, A., M. Oloso, M. Elshafei, A. Abdulraheem, and A. Al-Majed (2011). Support Vector Regression and Functional networks for Viscosity and Gas/Oil Ratio Curves Estimation. International Journal of Computational Intelligence and Applications. Vol. 10, No. 3. Sep. 2011. Pp 269-293.
- M.A. Al-Marhoun, S. Nizamuddin, A.A. Abdulraheem, S. Shujath Ali, A.A. Muhammadain. (2012). Prediction of crude oil viscosity curve using artificial intelligence techniques. Journal of Petroleum Science and Engineering. 86-87 (2012) 111–117.