The current development of unconventional resources (such as shale gas, shale oil, and tight sands) requires hydraulic fracturing, which involves injecting fluid at high pressure into the subsurface reservoir. Such injections (or fluid production) cause stress changes in the reservoir. These stress changes often result in failure of the rocks with a concurrent release of seismic energy as seismic waves. The nature of seismic wave propagation in the subsurface media is complex. Based on the direction of propagation and the particle motion, body waves can be classified into P-waves (primary, compressional wave) and S-waves (secondary, shear wave). Passive microseismic monitoring is based on recording these emitted waves and then using signal processing to extract characteristics such as amplitude, polarity, and arrival time, from which it is then feasible to estimate the location and character of the failure events ( McClellan, Eisner, Liu, Iqbal, Al-Shuhail and Kaka, 2018).
Passive microseismic monitoring requires new approaches to accurately detect and localize passive microseismic events in order to track the creation of fractures during and after the simulation and to evaluate the effect of the reservoir simulation. However, microseismic events usually have a very low signal-to-noise ratio (SNR), especially when monitoring sensors (receivers) are located at the surface where coherent and non-coherent noise sources are overwhelming. Therefore, we’re actively researching in enhancing the SNR of the monitoring event that will improve the monitoring process over the reservoir.
Over the past few years, we have introduced several new approaches to enhance, detect, and localize the passive microseismic event.
They include the following, but not limited to:
- Enhancement of passive microseismic events using the seismic interferometric approach to enhance detection of weak, passive seismic events
- Passive microseismic events enhancement in sensor arrays using autocorrelation based filtering
- Automated SVD filtering of time-frequency distribution for enhancing the SNR of passive microseismic events
- A new first-break picking algorithm that employs concepts from seismic interferometry and time-frequency (TF) analysis for passive microseismic events
- Enhancement of passive microseismic events using tensor decomposition and time-frequency representation
- Passive microseismic events enhancement and detection in sensor arrays using autocorrelation-based filtering
- Detection and denoising of passive microseismic events using time-frequency representation and tensor decomposition
- Observation-driven method based on IIR Wiener filter for passive microseismic data denoising
- Automatic microseismic event detection using constant false alarm rate processing in time-frequency domain
- Blind noise estimation and denoising filter for recovery of passive microquake signals
- Detection, location, and source mechanism determination with large noise variations in surface microseismic monitoring
Moreover, passive microseismic events are a useful indicator of fluid migration associated with hydraulic fracturing. Thus passive microseismic event monitoring has made significant contributions to understanding the geometry of fractures associated with hydraulic stimulation. The key is to track the creation of fractures during and after the stimulation.
Since 2013, we have been active in processing and analyzing passive microseismic events, generated during a multi-stage stimulation experiment, at a shale gas reservoir in Saudi Arabia. We aim to analyze microseismic events to better understand fracture growth and the role of pre-existing fractures during multistage stimulation.
At CPG we have been able to establish a Passive Microseismic Data Analysis Center including purchasing both hardware and software to analyze passive seismic data as well as training of several faculty members and students on processing, enhancing microseismic data. The equipment, software, and knowledge gained so far are extremely beneficial for the Department of Geosciences at KFUPM. We are now in a position to process and enhance passive seismic data and its application in training Saudi students for their future careers in the oil and gas industry.
The research team includes Dr. SanLinn I. Kaka, Prof. Abdullatif Al-Shuhail, and Dr. Umair bin Waheed from CPG and Prof. Azzedine Zerguine and Dr. Naveed Iqbal from the Electrical Engineering Department.
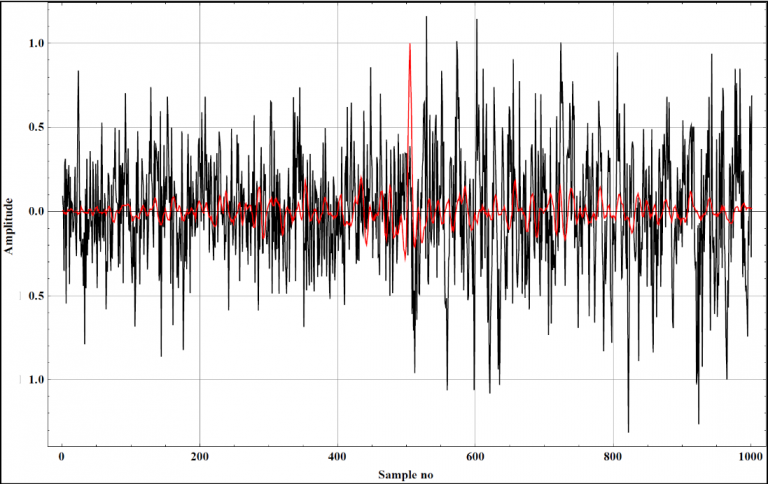
Comparison between the first traces of the enhanced (red) and raw records after adding noise (black).
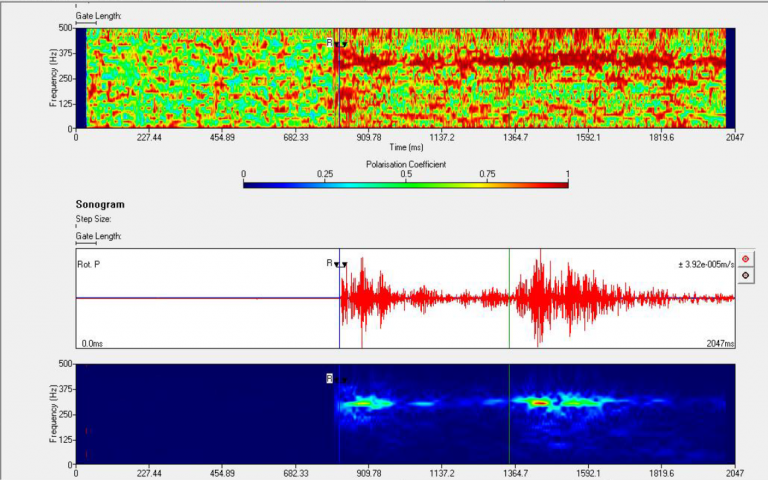
Example waveform from a ‘Located microseismic event’ a) Waveforms recorded in the 10 geophone tools, b) Polarisogram, and frequency content of an example component.
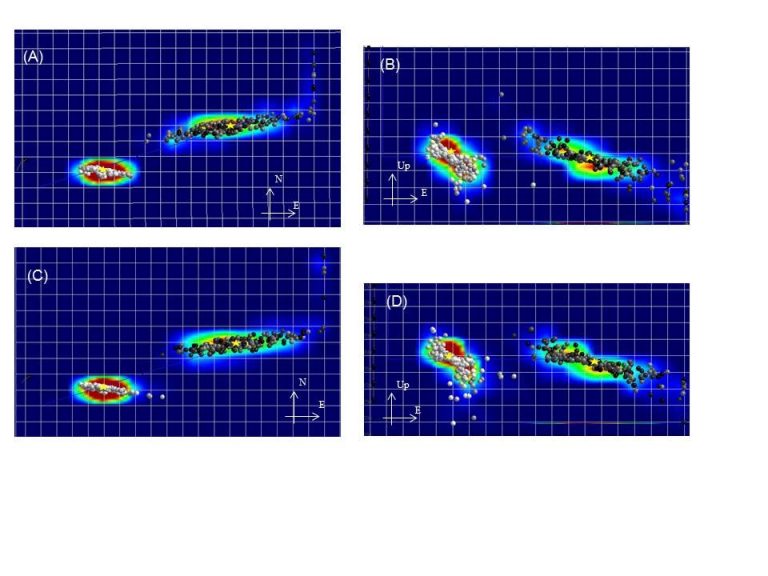
Contour density plots showing the results from the Monte Carlo simulation to analyze the sensitivity of event location against uncertainties in the velocity model. Location inversion was performed 200 times at each theoretical position using a velocity model in the inversion with a 5% and a 10% deviation from the theoretical model. The plots show the resulting event locations from each of the simulations; the results for simulations with 5% deviation are shown in panels A) map view, B) side view looking north and the results for simulations with 10% deviation are shown in panels C) map view and D) side view looking north. Yellow stars mark the true location for theoretical events located at an early stage (east side of the plots) and a late-stage (west side of the plots). Dark grey events correspond to the resulting locations for the simulations at the early position and light grey events correspond to the locations for the simulations at the late stage position. Grid spacing is 100 ft.
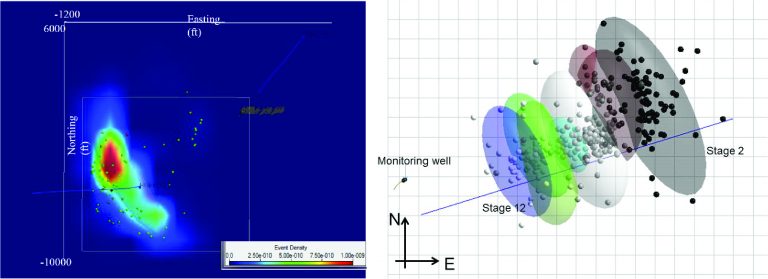
Locations of 1,294 microseismic events shown as density of events, b) Ellipsoids fitted to the located microseismic events, induced in each treatment stage, to determine the stimulated volume and fracture network geometry for each stage
References:
- Dmitry Alexandrov, Leo Eisner, Umair bin Waheed, SanLinn I. Kaka, and Stewart Alan Greenhalgh (2020), “Detection, location and source mechanism determination with large noise variations in surface microseismic monitoring”, Geophysics (Accepted).
- N Iqbal, B Liu, M Deriche, A Al-Shuhail, SL Kaka, A Zerguine (2019), “Blind noise estimation and denoising filter for recovery of microquake signals”, Exploration Geophysics 50 (5), 502-513.
- N Iqbal, A Zerguine, SL Kaka, A Al-Shuhail (2018), “Observation-driven method based on IIR Wiener filter for microseismic data denoising”, Pure and Applied Geophysics 175 (6), 2057-2075
- N Iqbal, E Liu, JH McClellan, A Al-Shuhail, SI Kaka, A Zerguine (2018), “Detection and denoising of microseismic events using time-frequency representation and tensor decomposition”, IEEE access 6, 22993-23006.
- JH McClellan, L Eisner, E Liu, N Iqbal, AA Al-Shuhail, SI Kaka (2018), “Array processing in microseismic monitoring: Detection, enhancement, and localization of induced seismicity”, IEEE Signal Processing Magazine 35 (2), 99-111.
- E Liu, L Zhu, AG Raj, JH McClellan, A Al‐Shuhail, SLI Kaka, N Iqbal (2018), “Microseismic events enhancement and detection in sensor arrays using autocorrelation‐based filtering”, Geophysical Prospecting 65 (6), 1496-1509.
- SanLinn I. Kaka, Juan, Reyes-Montes, Abdullah Al-Shuhail (2017), “Analysis of Microseismic Events during a Multi-stage Hydraulic Stimulation Experiment at a Shale Gas Reservoir”, Petroleum Geoscience 23 (386).
- N Iqbal, AA Al-Shuhail, SLI Kaka, E Liu, AG Raj, JH McClellan, (2017), “Iterative interferometry-based method for picking microseismic events”, Journal of Applied Geophysics 140, 52-61.
- N Iqbal, AA Al-Shuhail, SLI Kaka, E Liu, AG Raj, JH McClellan (2016), “Automated SVD filtering of time-frequency distribution for enhancing the SNR of microseismic/microquake events”, Journal of Geophysics and Engineering 13 (6), 964-973.
- A Al‐Shuhail, SLI Kaka, M Jervis, “Enhancement of passive microseismic events using seismic interferometry”, Seismological Research Letters 84 (5), 781-784.