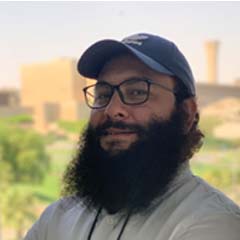
Ahmed Al-Sabaa
Drilling Lab Specialist, Laboratory Technical Support & Services
Focused and results-oriented professional with relevant experience in oil and gas drilling operations. Possess aptitude in coordinating with rig crews, and utilizing equipment to drill directional wells and to conduct training in well control & HSE for different oilfield personnel.
Gained great analytical skills to detect gaps in research and construct scientific methodologies to tackle problems using new artificial intelligence techniques along with lab experiments and data science. Has a proven experience in scientific research by a good number of published journal papers beside conference papers and presentations.
Lecturer for undergraduate and graduate drilling engineering courses in highly ranked university. Recognized as an expert in drilling engineering topics and assisting professors in building new curriculums in addition to reviewing and improving courses to update them with the latest results and discoveries.
Educational Qualification
- M.S., Petroleum Engineering, King Fahd University of Petroleum & Minerals – KFUPM.
- B.S., Petroleum Engineering, Suez University.
Research Interests
- Well Control
- Artificial Intelligence Applications
- Drilling Engineering
Selected Publications
- Alsabaa, A., & Elkatatny, S. (2021). Improved Tracking of the Rheological Properties of Max-Bridge Oil-Based Mud Using Artificial Neural Networks. ACS Omega, acsomega.1c01230. https://doi.org/10.1021/acsomega.1c01230
- Alsabaa, A., Gamal, H. A., Elkatatny, S. M., & Abdulraheem, A. (2020a). Real-time prediction of rheological properties of all-oil mud using artificial intelligence. 54th U.S. Rock Mechanics/Geomechanics Symposium.
- Alsabaa, A., Gamal, H.A., Elkatatny, S. M., & and Abdulraheem, A. (2020). Real-Time Prediction of Rheological Properties of All-Oil Mud Using Artificial Intelligence. American Rock Mechanics Association. https://doi.org/https://www.onepetro.org/conference-paper/ARMA-2020-1645
- Alsabaa, A., Gamal, H., Elkatatny, S., & Abdelraouf, Y. (2022). Machine Learning Model for Monitoring Rheological Properties of Synthetic Oil-Based Mud. ACS Omega. https://doi.org/10.1021/ACSOMEGA.2C00404
- Alsabaa, A., Gamal, H., Elkatatny, S., & Abdulraheem, A. (2020c). Real-time prediction of rheological properties of invert emulsion mud using adaptive neuro-fuzzy inference system. Sensors (Switzerland), 20(6). https://doi.org/10.3390/s20061669
- Alsabaa, A., Gamal, H., Elkatatny, S., & Abdulraheem, A. (2021). New correlations for better monitoring the all-oil mud rheology by employing artificial neural networks. Flow Measurement and Instrumentation, 101914. https://doi.org/https://doi.org/10.1016/j.flowmeasinst.2021.101914
- Alsabaa, A., Gamal, H., Elkatatny, S., & al Shehri, D. A. (2022). Rheology Predictive Model Based on an Artificial Neural Network for Micromax Oil-Based Mud. Arabian Journal for Science and Engineering. https://doi.org/10.1007/S13369-022-07123-7
- Mahmoud, A. A., Elkatatny, S., Alsabaa, A., & Shehri, D. al. (2020). Functional neural networks-based model for prediction of the static young’s modulus for sandstone formations. 54th U.S. Rock Mechanics/Geomechanics Symposium, 1–8.
- Salem, A. M., Attia, M., Alsabaa, A., Abdelaal, A., & Tariq, Z. (2022a). Machine Learning Approaches for Compressibility Factor Prediction at High- and Low-Pressure Ranges. Arabian Journal for Science and Engineering. https://doi.org/10.1007/S13369-022-06905-3